Break Into Data Science: The Definitive Guide [2022 Update]
Lessons Learned From Interviewing 16 Data Scientists Who Transitioned Into Data Science , So You Can Do The Same in 2022
Table of Contents:
- Do You Need to Go Back-to-School for Data Science? Maybe Not!
- Using the Build-Measure-Learn Process for Landing Data Science Jobs
- Advice for Current Students Trying to Break Into Data Science
- Ways In Which People With Non-Traditional Backgrounds Have An Advantage
- Advice for Data Analysts Transitioning Into Data Science
- How To Stay Motivated Self-Learning Data Science
- Be Careful With Yak Shaving
- Why Doing Real-World Data Science Projects Is So Crucial
- Recommended Online Data Science Courses
- Best Ways To Learn Coding for Data Science
- R vs. Python vs. Matlab - What Programming Language You Should Learn for Data Science
- How To Learn the Math Behind Data Science
- Recommended Data Science Bootcamps
Data Science Is Dope, And So Is Diversity
I wish I had a more articulate way of saying this, but I don’t.
Data Science Is SO FREAKING Dope!
From Netflix recommendations to the Facebook feed, data-driven products are the backbone of the world’s most cutting edge tech companies. In the public sector, Data Scientists are at the forefront of modeling and fighting COVID-19. On Wall Street, Data Scientists are helping to create more efficient capital markets and unlocking hundreds of billions of dollars worth of hidden value through mining alternative data. Data Scientists not only get to solve society’s toughest and most interesting problems all day, but also get paid very well for it too!
The average base salary for a Data Scientist in the US is $123,000, according to Indeed. At a top tech company like Facebook, the median compensation for a Senior Data Scientist is $253,000 per year between salary, stock, and bonus, according to Levels.FYI. These salaries aren’t that rare either! A search on LinkedIn reveals that 3,600 people currently hold the title “Data Scientist” at Facebook.
Given how intellectually stimulating and damn lucrative Data Science is, it’s no wonder that Harvard Business Review in 2012 called “Data Scientist” the hottest job of the 21st Century – 10 years later in 2022 we still think it's pretty true!

Unfortunately, breaking into Data Science isn’t super easy. Doubly so for those coming from non-traditional backgrounds.
We wrote this guide because we believe improving access to information and bringing visibility to people who pursued non-traditional paths into Data Science will lead to more diverse voices joining this critical field.
Because we, the authors of this guide, have pretty traditional backgrounds, we made sure to interview Data Scientists with a variety of different experiences to learn how they broke into Data Science.
We interviewed 16 diverse people who previously were Data Analysts, Mechanical Engineers, Economists, Neuroscientists who are now Data Scientists at the world's top tech companies (FANG) and Wall Street firms. Some have PhDs, some just have undergraduate degrees. Some are self-taught, some did bootcamps, and some went back to school to learn Data Science.
We put together their stories and their tips to create the most definitive guide to breaking into Data Science on the internet.

About This Guide's Authors

Kevin Huo previously worked as a Data Scientist at a Hedge Fund. Before that, he was a Data Scientist at Facebook working on the Facebook Groups product. Kevin graduated from the University of Pennsylvania with a degree in Computer Science and a degree in business from the Wharton School with concentrations in Economics, Finance, and Statistics. While in college, he interned at Facebook, a large hedge fund ($16B AUM), and Bloomberg.
Nick Singh previously worked at SafeGraph - a Data-as-a-Service startup where he managed their data journalism initiative and ran product marketing for SafeGraph’s geospatial datasets. Before that, Nick was a Software Engineer on Facebook’s Growth Team, and interned at Google’s Nest Labs on the Data Infrastructure Team.
Together we wrote Ace the Data Science Interview, a #1 best-seller on Amazon, and created the video course Ace the Data Job Hunt!
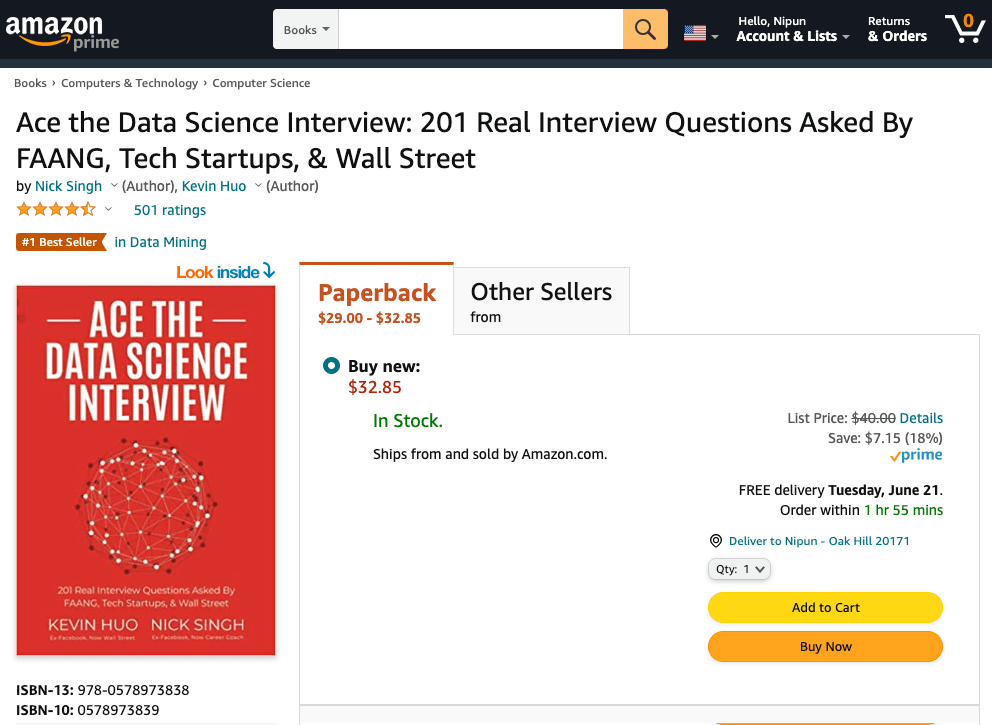
Breaking Into Data Science In 2022; Advice for People Currently In The Workforce Or School
By talking with the sixteen Data Scientists from various backgrounds, we leveraged the wisdom of the crowds to answer the top questions people had about transitioning into Data Science in 2022.
In this section, we address questions like:
- Do you need to go back to school and get a formal degree in Data Science?
- How do you land Data Science jobs via an intermediate stepping stone job?
- How to apply the iterative Build-Measure-Learn approach to Data Science job hunting
- How can college & grad students break into Data Science?
- What should people from non-traditional backgrounds keep in mind when breaking into Data Science?
- How do you transition from Data Analyst to Data Scientist?
General Framework For Deciding Whether To Go Back To School For Data Science
Nick’s co-worker, Ryan Fox Squire, has worked as a Data Scientist at SafeGraph for several years and before that was a Data Scientist at Lumosity (the brain training game). He dropped out of a PhD in Neuroscience at Stanford to pursue Data Science. When asked whether it makes sense to go back to school for data science, his advice is as follows:
“You should only go to school as a last resort. Going to a Masters program for 1–2 years (or a PhD program for even longer) is a huge investment of time and money. Learning on the job, accumulating work experience, and self-teaching are much preferable, if you can manage it. Put another way— don’t go back to school until you’ve gathered sufficient evidence that going back to school is absolutely necessary to achieve your dreams.” - Ryan Fox Squire on Quora
Overwhelmingly, the Data Scientists we surveyed agreed that much of the learning happens on the job. So the best way to learn Data Science is to join a company and be a Data Scientist. I know, sounds like a catch-22! But it’s not, thanks to stepping-stone jobs!

Using Stepping Stone Jobs To Break Into Data Science
Stepping stone jobs are roles which have you doing some work related to Data Science, or working closely with Data Scientists but aren’t jobs in which you are a full-on proper Data Scientist.
Data Analyst, Software Engineering, and Business Analyst roles often serve as great stepping-stone jobs that can help you solve the catch-22 of “I need Data Science work experience to become a working Data Scientist.” And unlike going back to school, you get paid in stepping-stone jobs while transitioning into Data Science!
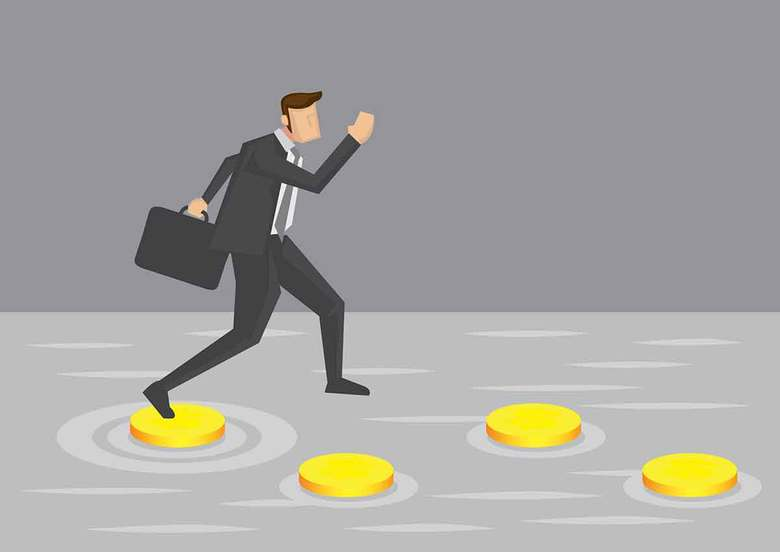
The people we interviewed who successfully used stepping-stone jobs to break into Data Science said the key was taking initiative. They recommended finding data-related side-projects which will help your company. Ideally, the projects you choose will force you to learn from Data Scientists within your organization. This is a great way to hack mentorship from a practicing Data Scientist - something most online-courses or degree programs lack.
After contributing enough value in the workplace through the stepping-stone job and the data side-projects, you can make the case to lateral into a Data Science job within the same company. Key here is to have your employer take a bet on you since that’s much more likely to happen than a random new employer making a bet on you.
Of course, using stepping-stone jobs requires a ton of work and self-learning on the side. Maybe going to school is the more comfortable choice, despite the opportunity costs. So, how do you know if you're already well suited for a job (or stepping stone job) in Data Science, and don’t need to go back to school? Apply the Build-Measure-Learn philosophy below.
The Iterative Build-Measure-Learn Process For Landing Data Science Jobs
We can borrow a page from the book, the Lean Startup, which popularized the method of “Build-Measure-Learn” for building a successful startup and apply it to building a career in Data Science.

Phase #1: The build phase: take an inventory of your skills, projects, and work experience. See if you can position any of what you have done or known as Data Science.
The regression analysis you did for your environmental science class? Data Science! That analysis of your company's revenues where you also forecasted it for the next 4 quarters? Data Science!
Once you’ve put together a Data Science focused resume, positioned your work into compelling portfolio projects, and brushed up on the fundamentals, it’s time for the “Measure” phase.
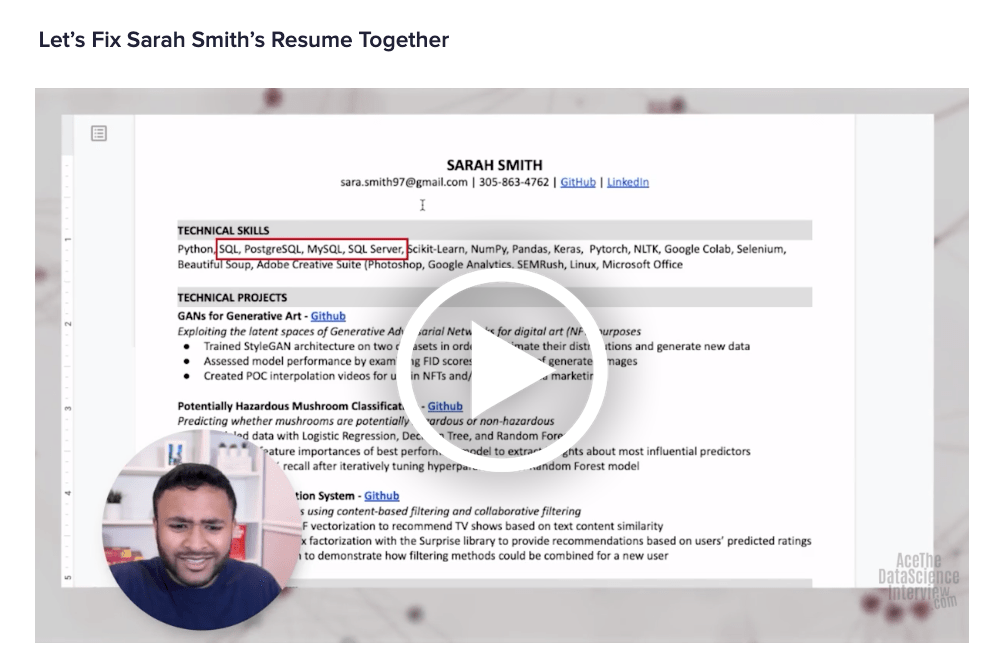
Phase #2: The measure phase is all about testing your viability as a Data Scientist. And the only way to test this is by applying to jobs, and measuring outcomes.
You should NOT go back to school until you’ve applied to a few jobs and seen what happened.
Did you get interviews? If yes, great - maybe you don’t need to go to school. And if not, are your portfolio projects okay? Have you tried cold-emailing recruiters and hiring managers instead of applying via online job portals,which are effectively blackholes for time and energy?
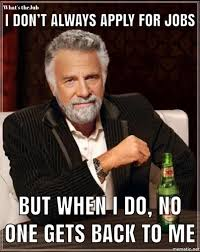
Applying online to jobs is effectively throwing your resume into a blackhole. Instead, use these eight cold-email tips to land your dream job. And watch the video below on 6 cold email rules you can't break, to get even more advice on how to cold email yourself to more interviews.

Got interviews, but failed them? Great, you're now ready for Phase 3.
Phase #3: The learn phase: due to failures in callbacks or interviews, you now know what skills to brush up on. Go practice more interview questions in Ace the Data Science Interview. Take a Coursera class, or do some reading on the topics you feel weak on. Then build a portfolio project in that area to deeply understand and apply what you learned.
Go through this loop a few times, and soon you’ll have built the skills, resume, and projects to potentially break into Data Science without needing to go to school. The big takeaway here is that some people don’t even apply to Data Science jobs and assume they are under qualified since they didn’t go back to school. In this way, you just disqualified yourself from a job without even applying. Not great!
So don’t hesitate to put yourself out there to get shot down a few times, before deciding you need to go back to school to learn Data Science. Now, if you are still in school, this next section is for you.
Advice for Current Students Trying to Break Into Data Science
Currently enrolled students are in luck. You likely have the most time to learn data science, take internships, and break into data science. There is a path to Data Science that doesn’t necessitate going to Grad School for it.
The Data Scientists we surveyed overwhelmingly recommended people to take as many statistics and Computer Science classes as possible. Courses on probability, statistics, linear algebra, and vector calculus will teach you the fundamental math needed for Data Science.
From the Computer Science side, taking classes on Data Structures, Algorithms, and Databases, and Python should be helpful for aspiring Data Scientists. If you have space, squeezing in Machine Learning classes is an excellent idea.

If you don’t have space for these foundational classes, or are already very deep into a Masters or Ph.D. in a different discipline, there is still a way to break into Data Science without directly taking these classes.
For example, if you are studying Economics, try to take as many econometrics classes as possible. Make sure you can re-implement the assignments in R or Python. For the popular Introductory Economics by Wooolridge textbook, you can find the companion guide “Using Python for Introductory Econometrics.” Similarly, if you are in Life Sciences, try to take biostatistics or bioinformatics classes.
See if your senior year capstone project, or masters thesis, can be done on a data-driven topic. Many of the Data Scientists we interviewed from non-traditional backgrounds, in Civil Engineering or Biology, ended up doing very data-driven thesis projects which is how they kindled their passion for Data Science and convinced employers they knew Data Science without the exact degree in it.
If you don’t have time to take any of these classes or do projects while enrolled in school, it might be worth delaying your graduation by a semester or two. With COVID-19 wreaking havoc on the economy, hiding out in school isn’t the worst option if financially feasible.
Delaying graduation by a semester might give you an extra summer to do an internship. And landing a Data Science internship goes a LONG way when it comes time to recruit for full-time Data Science roles. You can also skip ahead to the “Self-Teach Data Science Resources” section if you can’t squeeze these classes.
Note Of Encouragement For People From Non-Traditional Backgrounds
Because of how multi-disciplinary Data Science is, your non-traditional background can actually be used as a strength. So much of real-world data science is using domain knowledge with simple models.
For example, at big tech companies, knowing how to make the product better is more important than doing super-advanced and complicated modeling. The modeling is just a tool to make a big impact on your product. So if you are transitioning from Product Management or User Experience Design into Data Science, you already have a leg up when interviewing at product-based tech companies.
So focus your job-search in the same industry you already have experience with and expect a nice boost when looking for Data Science jobs.
Advice For Data Analysts Transitioning Into Data Science
Data Analysts transitioning into Data Science have the easiest and hardest transition to make. You are so so close. But unfortunately, people will gatekeep and say “no, you're not a real data scientist”. Even if you are working on data all day everyday!
Here’s the psychology behind why this transition is so hard to make. Mechanical Engineers transitioning into Data Science are unpriced assets. We don’t know if they are good or bad at Data Science because they were in a different field. Employers see that there is some uncapped upside left and decide to give the Mechanical Engineer a chance.
But if you are already a data analyst, in a way, you’re a known commodity. This is how you end up getting pigeonholed into another data analyst job, even if you know more Data Science than someone transitioning into Data Science from Mechanical Engineering.
Yes, I know it’s messed up and unfair but we wanted to call this point out for people struggling with the transition from Data Analyst to Data Science.
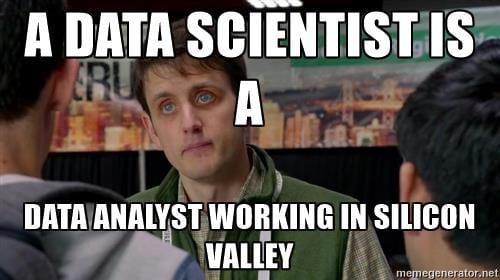
So if you are a Data Analyst trying to become a Data Scientist, over-index on your technical skills. Go study this list of 17 must-read Data Analytics books. While Deep Learning and Machine Learning are overhyped, you might need to do some of this in your projects since you are trying to overcome the stigma that you are “just a Data Analyst”.
So go Play around with AWS. Use GitHub for source control. Up-level your Python coding ability by practicing Python Coding Interview Questions. Show the interviewing company you are genuinely a Data Scientist, maybe even a Machine Learning Engineer, just accidentally stuck in a Data Analyst role.
High-Level Advice To Learn Data Science
Before we dive into the best courses, bootcamps, and certifications to learn Data Science, we believe starting with some meta-thoughts on learning Data Science is essential.
We cover:
- How to Overcome the #1 Problem People Faced When Studying Data Science – Losing Motivation
- How Not to Get Overwhelmed When Learning Data Science
- Do Not Yak Shave!
- Why Portfolio Projects Are Crucial for Data Science
It’s all About Motivation Management; Do Yourself A Favor And Make It Fun
When we asked the panel of Data Scientists, “what do you think separated you from people who did NOT successfully break into Data Science,” most mentioned some form of “not giving up”.
They said unsuccessful peers had the mental ability for Data Science, but at some point in the process they gave up and lost motivation to keep going.
The number #1 way we know to keep people motivated during this tough task of learning Data Science is to make sure you are having fun with what you are learning and solving problems you care about.
So if you are into art, design, or journalism choose books like “Storytelling with Data: A Data Visualization Guide for Business Professionals” to create superior graphics and stories with data.
Or try the book “Practical SQL: A Beginner's Guide to Storytelling with Data” written by WSJ Data Journalist Anthony DeBarros which offers a very practical guide to going from zero to hero in SQL, and tons of applications of SQL to find interesting trends and stats in data for data journalism use cases.
Are you into health and medicine? Give this Coursera specialization on Statistical Analysis with R for Public Health Specialization a try. Love basketball? Use datasets from the NBA in your portfolio projects. Love music? Go classify songs into genres based on their lyrics.
Tired of studying SQL from boring? Give these 3 fun SQL learning games a try.

Don’t Get Overwhelmed By How Much There Is To Learn
Another reason people quit when learning Data Science is that they get overwhelmed with how much there is to learn. But good news: there is less than you think there is to learn.
When you look at job descriptions, you’ll often see a myriad of skills required. But a secret amongst expert job-seekers is that most job requirements are optional. With 90% of job descriptions asking for unreasonable qualifications, and with a shortage in qualified Data Scientists, it’s possible to get roles where you only meet half the “requirements”.
Another reason there is likely less to learn than you think: practicing Data Scientists apply a fraction of what is taught in a Data Science degree program. At a company like Facebook, having domain expertise, good data intuition, great SQL skills, and strong statistical fundamentals should be good enough.
While knowing the intricacies of deep reinforcement learning might help...most Data Scientists at a company don’t need to know that. And even if Deep Learning is needed, companies like Facebook and Google have an army of PhDs in Computer Science to work on it rather than the average Data Scientist.

Be Careful Of Yak Shaving
In Software Engineering, there is a popular phrase: “yak shaving”. You are feeling cold, so you need a sweater and don’t have one, and then you follow the logical sequence of steps and soon find yourself shaving a yak for wool.

When it comes to learning Data Science, it’s easy to get lost in all the math, complexity, advanced techniques, and strive for perfection. Don’t fall into this trap!
So much real world Data Science is simply about cleaning the data, using straightforward tools like linear regression, and presenting the analysis in a convincing way. So focus first and foremost on learning enough to solve the problems you face at the workplace or when doing your portfolio project.
It’s too easy to think that solving a problem necessitates Deep Learning, which then means you need to understand Neural Nets, which means you need to take a 1-week refresher of Linear Algebra. You’ll burn yourself out going down the endless rabbit hole.
Why Doing Real-World Data Science Projects Is So Crucial
At the end of the day, after you learn the basics from courses or books, the real-world learning happens by tackling real world Data Science projects.
Not by repeating the trite MNIST handwriting recognition project, or the overdone IRIS Sepal length classification project.
If you are already in the work-force, see if you can do side-projects at your company as we mentioned in the “A General Framework For Deciding To Go Back To School For Data Science” section.
And if you aren’t in the workforce, and need inspiration on how to find and build exciting Data Science projects, make sure to read Nick’s guide on “Creating Kick-Ass Data Science & ML Portfolio Projects”.
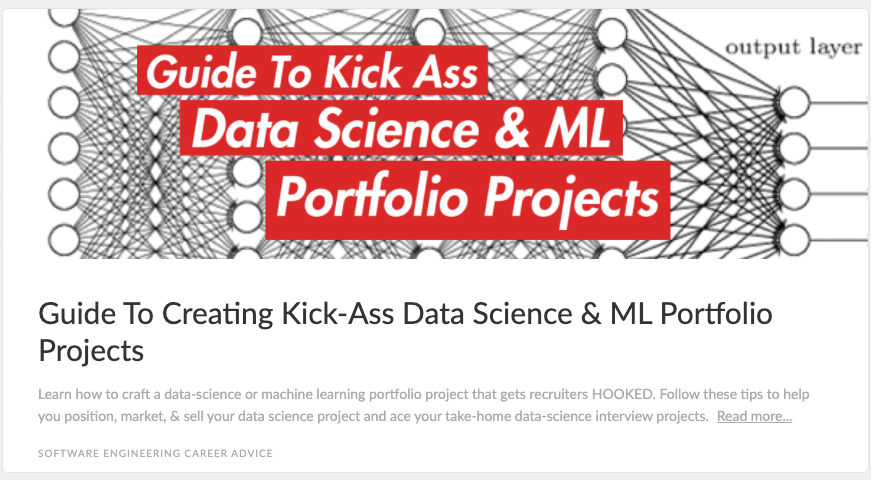
For a concrete example of 6 dope portfolio projects that recruiters will love, including one that uses Data Science to get more Tinder dates, watch the video below, which is a free preview from Nick's Ace the Data Job Hunt video course:

The Best Courses, Bootcamps, and Certifications To Learn Data Science
We surveyed 16 Data Scientists, along with many students at different stages of learning Data Science, to crowd-source some recommendations on learning Data Science.
We put together
- General Recommendations for Online Data Science Courses
- Best Ways to Learn Coding for Data Science
- Advice on Which Programming Language to Learn for Data Science
- How to Learn the Math Behind Data Science and Machine Learning
- What Some People’s Favorite Data Science Bootcamps Were
- Data Science Certifications (Which Likely Aren't Worth It!)
General Recommendations For Online Data Science Courses
Learning SQL is KEY! I made a 30-day SQL learning roadmap, which covers free resources that'll take you from SQL zero to SQL hero.
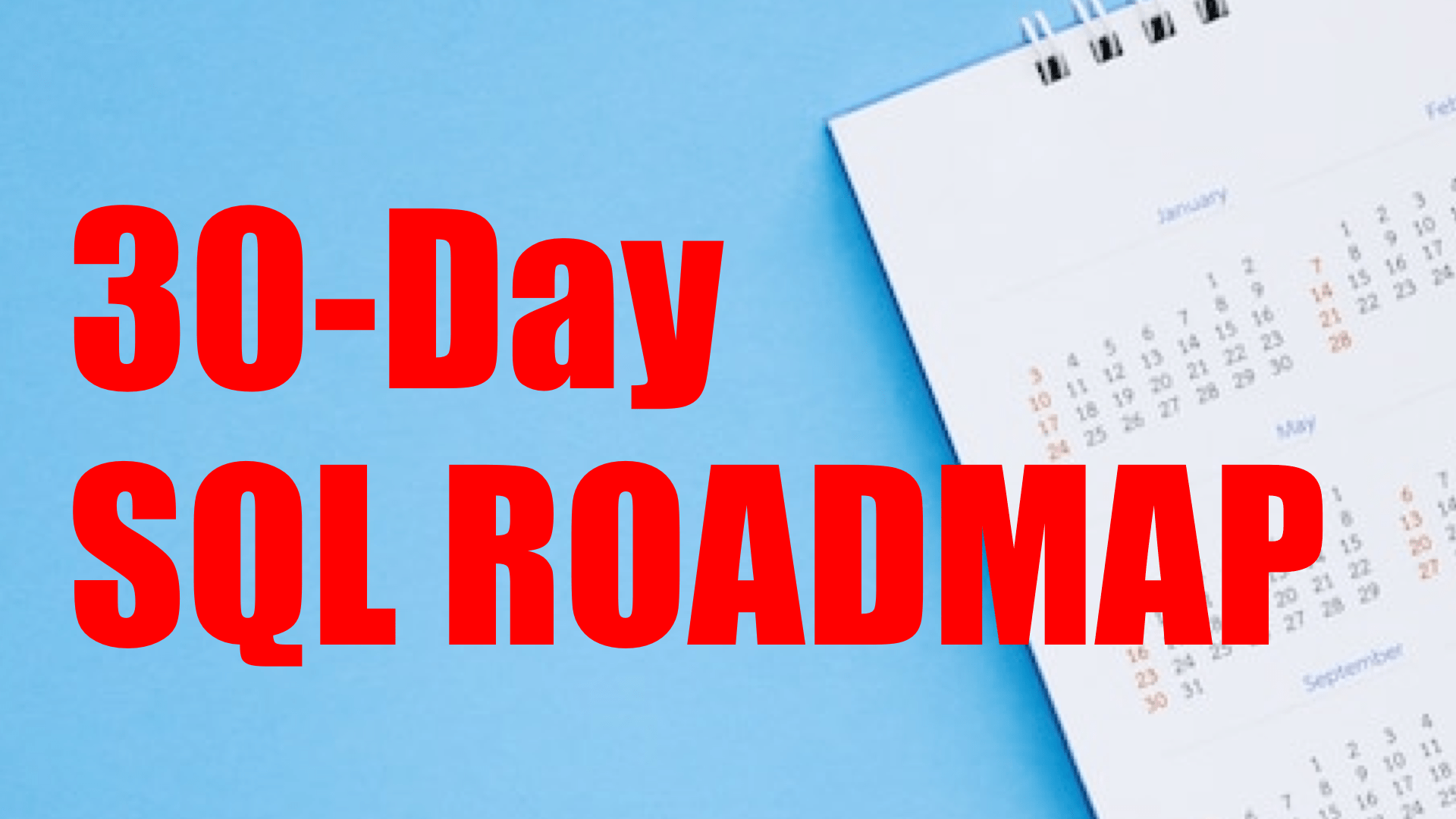
Word of advice: Once you learned the basics, start practicing SQL Interview questions on DataLemur!
For a broad survey and intro, try Harvard’s CS109 Introduction To Data Science class, taught in Python. It teaches from more of an ML, modeling perspective.
For a course grounded more in an inferential and foundational statistics-based approach, try EdX - UC San Diego’s Probability and Statistics in Data Science using Python. Doing this course should help you ace these 40 Statistics and Probability interview questions.
For a more applied approach, taught in R, you can try MIT’s “Data Analysis for Social Scientists” on EDX. This is a good fit if you don’t have a strong statistical background or already know R.
There’s also the famous Andrew Ng Coursera course on Machine Learning. This is taught in Matlab/Octave - a good choice if you know either of those languages, but not perfectly ideal if you don’t.
Kirill Eremenko’s Data Science A-Z on Udemy also has received rave reviews. It uses Tableau, Excel, and SQL Server - an excellent choice for Data Analysts and BI professionals who may already be familiar with these tools.
For programmers who already have a good grasp of Python, and some of the basics for Data Science, the book Hands-On Machine Learning with Scikit-Learn and TensorFlow offers a great mix of theory and practical application. Also is a great second course to take for Machine Learning aspirants who have taken one of the earlier Python-based Data Science courses mentioned above.
For more book lovers, we came up with a comprehensive list of the 13 best Data Science books – highly recommend you read these too if you're serious about leveling up!
Best Ways To Learn Coding For Data Science
Many of the people who transitioned into Data Science struggled with the programming aspect of Data Science. Having strong Software Engineering fundamentals is one of the best things you can do to get leverage in your Data Science career. This is because so much because real-world data science involves
- data cleaning and munging
- pulling in multiple data sources via APIs
- setting up recurring pipelines and scripts to process data
- retrieving data from databases with SQL (here's a realistic SQL example from Facebook)
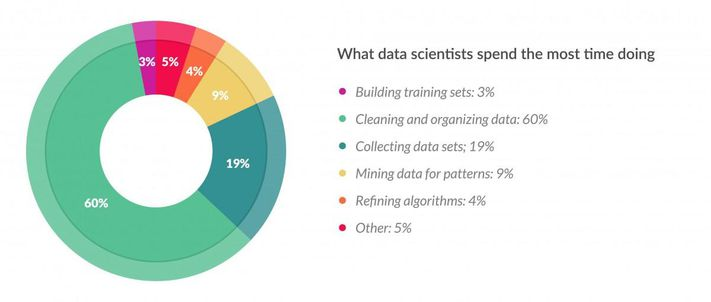
Software Engineers will already be familiar with doing the tasks mentioned above. But people with weak programming skills will struggle on these foundational parts of real-world Data Science.
If you are a complete noob at programming, a book like Automate the Boring Stuff with Python: Practical Programming for Total Beginners is a great place to start. It keeps learning Python practical, engaging, and project-focussed.
This is a great step before working through a Python focussed Data Science class. A more intermediate/advanced book to go through for strong programmers is Python for Data Analysis: Data Wrangling with Pandas, NumPy, and IPython, which is all about the data cleaning & manipulation aspect of Data Science. It’s written by Wes McKinney - the founder of the Python pandas package. This should help with the plethora of data cleaning problems you’ll run into doing real world Data Science.
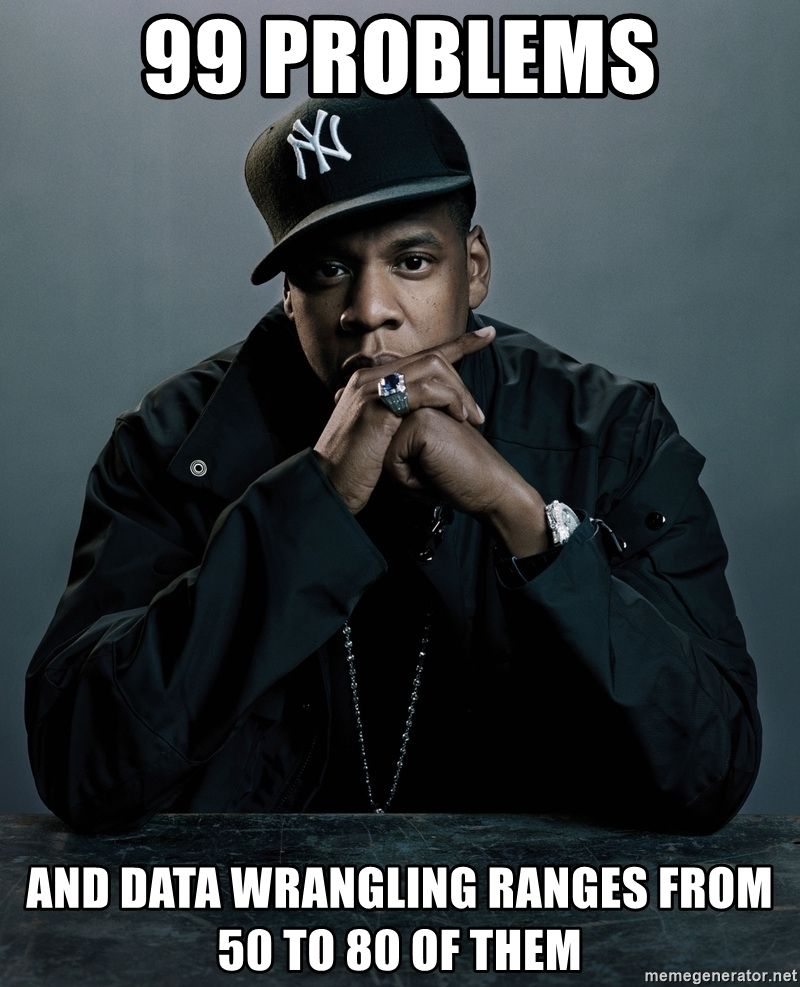
What programming language should I learn for Data Science?
If you know Python, you are set. Due to the plethora of Data Science packages, and Python’s use outside of Data Science in fields like Machine Learning, Data Engineering, and Software Engineering, Python is our favorite programming language for Data Science.
If you know programming languages like C++, Java, or Javascript, learning Python should be easy.
If you know R, go after learning more Data Science in general. If you HAVE to learn Python for a project or job, then obviously do it. But you can get very very far as a Data Scientist with R so it’s not bad to maintain momentum and keep using this tool you already know.
If you are using SPSS, Stata, or Matlab, and are trying to become a proper Data Scientist, go learn Python. Else, it’s not bad to stick with what you know for your first or second class in Data Science. But you’d likely want to switch over at some point as you progress, and when you do Python is your best bet.
And if learning Python is intimidating, maybe start by learning SQL – it's much easier to ramp up on than Python/R. Plus, SQL interview questions show up all the damn time in Data Analytics interviews, so maybe skip out on Python if your a total noob to Analytics.
How To Learn the Math Behind Data Science
People who already knew some coding or scripting, like web developers, said their most significant issue was learning the math behind Data Science. They knew how to import libraries like scikit- learn and OpenCV in Python, but didn’t know what techniques or tools to apply when. They can make random Neural networks with Keras...but had no idea what was happening behind the scenes.
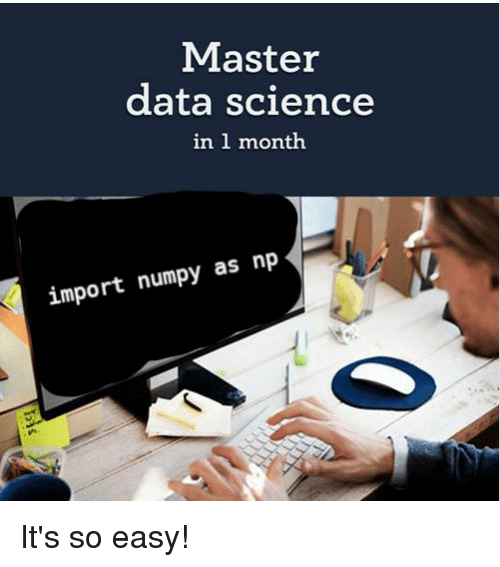
Programmers without a stats background “doing Data Science”
The most essential math behind Data Science is probability, statistics, multivariate calculus, and linear algebra. Calculus and linear algebra becomes more important for Machine Learning aspirants, but can be skipped during your first-pass when learning Data Science. This Coursera specialization is a good place to start. Khan Academy is also an option if you want to quickly jump in and refresh some concepts.
If you take a second pass, and you’ve refreshed your understanding of college linear algebra and calculus, you can go after the classic The Elements of Statistical Learning: Data Mining, Inference, and Prediction or Bishop’s Pattern Recognition and Machine Learning. These books are great for Machine Learning aspirants. But if these books are too difficult to get through, don’t fear.

As mentioned, Machine Learning is just one set of tools in the Data Science toolbox. Working through these texts can be overkill for entry-level Data Science jobs, and it can be higher ROI spending time working on real-world projects instead of working through tough machine learning textbooks. The best way to assess your skills, and see how you shape up against others, is by trying these real Machine Learning Interview Questions on DataLemur.
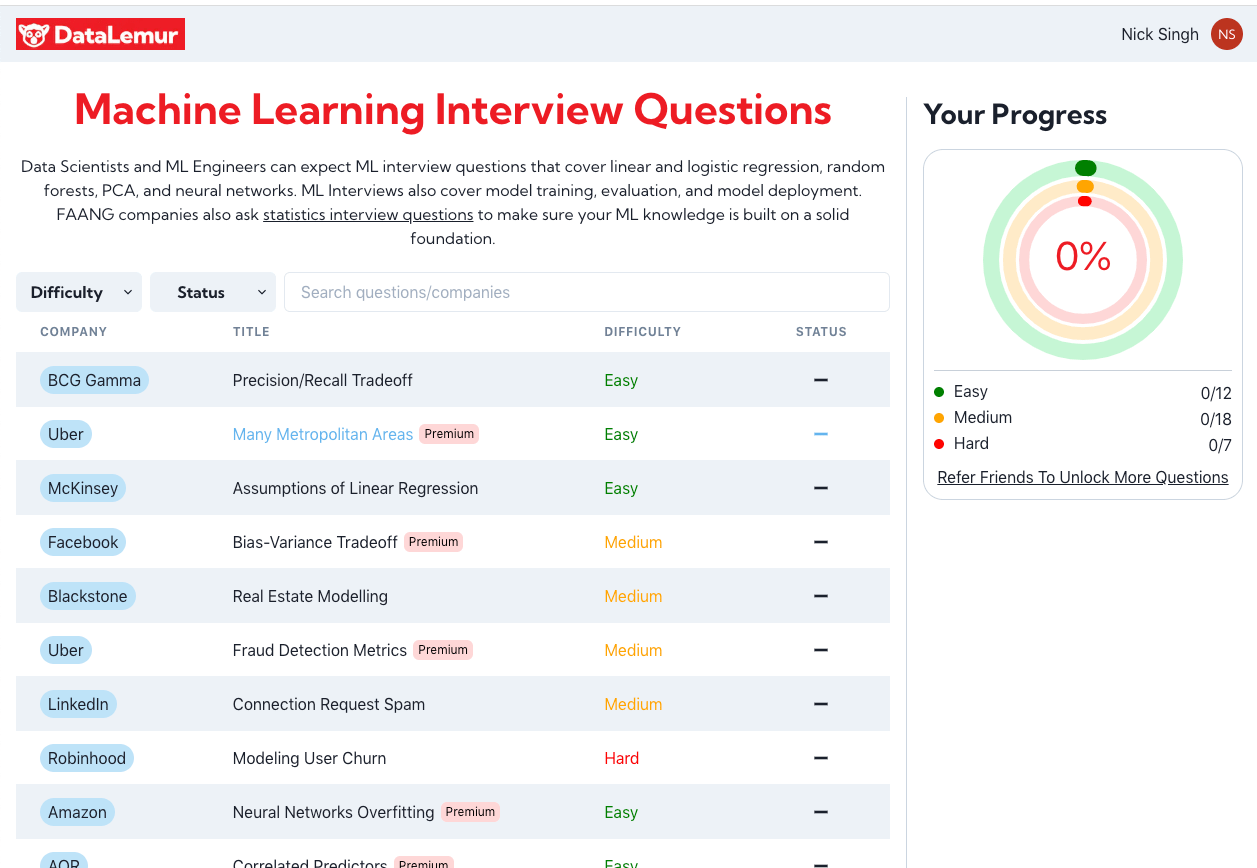
Data Science Bootcamps
Many of the Data Scientists we surveyed who did not have degrees in relevant fields like Statistics or Computer Science went to Bootcamps to learn. While the material was high-quality, they said that the real reason to pay for a bootcamp is being in a cohort of other motivated people slogging through the same material you are. The underlying content could be found at other places, but the accountability and cohort camaraderie made Bootcamps a great way to structure learning on a tight timeline.
Most traditional bootcamps have a few things in common. There’s often an application process (comprised of interviews, technical challenges, discussion of your experience), . With the COVID situation, most have an online option, though in regular circumstances many of them also offer in-person instruction. The bootcamps aim to do three things: give you a good foundation in data science skills, help you build a portfolio, and provide career advice and mentorship.
Some recommended bootcamps that the people we surveyed, or the friends of the people we surveyed, recommended:
- Insight Fellows Program is a 7 week, well-reviewed program but is for graduate-level students who have more of the academic backgrounds (stat, probability, research) and are looking to bridge the gap between academia and Data Science as a career
- NYC Data Science Academy is a 12-week bootcamp that goes in-depth with basic data science tools while also covering complementary technical skills that will be added-value in a data science role. There is an application/interview process for getting into the bootcamp - so this option may be better suited for people with technical backgrounds or PHD/Masters students studying something data-related. During the 12-weeks, participants complete at least four projects that they showcase and can put in their portfolio, while also receiving 1-on-1 career mentoring. If you prefer to do something less costly and shorter, NYC Data Science Academy does offer shorter courses that are on a specific topic, such as Machine Learning with Python or Data Visualization with R
- Metis and FlatIron are very similar to NYC Data Science Academy in terms of duration, application process, content and cost.
- General Assembly has similar prerequisites (expectation of Python proficiency, basic statistics and programming) with one twist - it’s self-paced and flexible with timing. Thus, you can theoretically work a full-time job while doing your coursework after work or on the weekends. Therefore, you have the option to do time-based tuition (paying per-month rather than for the full-course upfront). With all that in mind, the total tuition if you do pay up-front with General Assembly is a lot cheaper than most bootcamps (about $4,000 vs the typical $16,000-$17,000)
- Galvanize has a similar application process but is more flexible with your level of experience - depending on your level of experience, they’ll adjust the amount of pre-bootcamp work for you. Galvanize also gives the option of an income-sharing-agreement (ISA) where you don’t pay the full tuition until after you’ve secured a job. This is more catered for people that aren’t masters/PHD students, and may not even have technical or data-oriented backgrounds
Data Science Certifications
Don’t pick a bootcamp or online course based on certification - actually take a closer look at the topics/content. Because honestly, most recruiters at top-tech companies and wall street funds don’t care about any Data Science certification.
Certifications don’t move the needle. Skills matter much more. That being said, certifications are not a bad way of keeping you motivated, structuring your learning, and offering you a way to test your skills at the end. We heard good things about IBM’s Data Science Professional Certificate.
In Conclusion: Steps To Landing Your Dream Job In Data Science
Putting all the tips together from the guide, here are the steps to break into Data Science.
- Make sure your coding skills are where they need to be
- Make sure you have enough statistics and probability background to understand which methods to apply when
- Make sure you’ve applied these skills to real-world portfolio projects. Even better if you got to apply these skills in a stepping stone job
- Put together your portfolio, clean up your resume, and start cold-emailing your way into jobs
- Repeat #4….and eventually you’ve broken into Data Science. OR…
- If you fail too many times with step #4, enroll in a degree program or bootcamp to structure your learning and keep you motivated. Finish that, then get back to step #4
Thank You For Reading - We Have More Data Science Guides On The Way!
Want more like this? Make sure you to grab a copy of Ace the Data Science Interview on Amazon, or join our free 9-day interview crash-course.
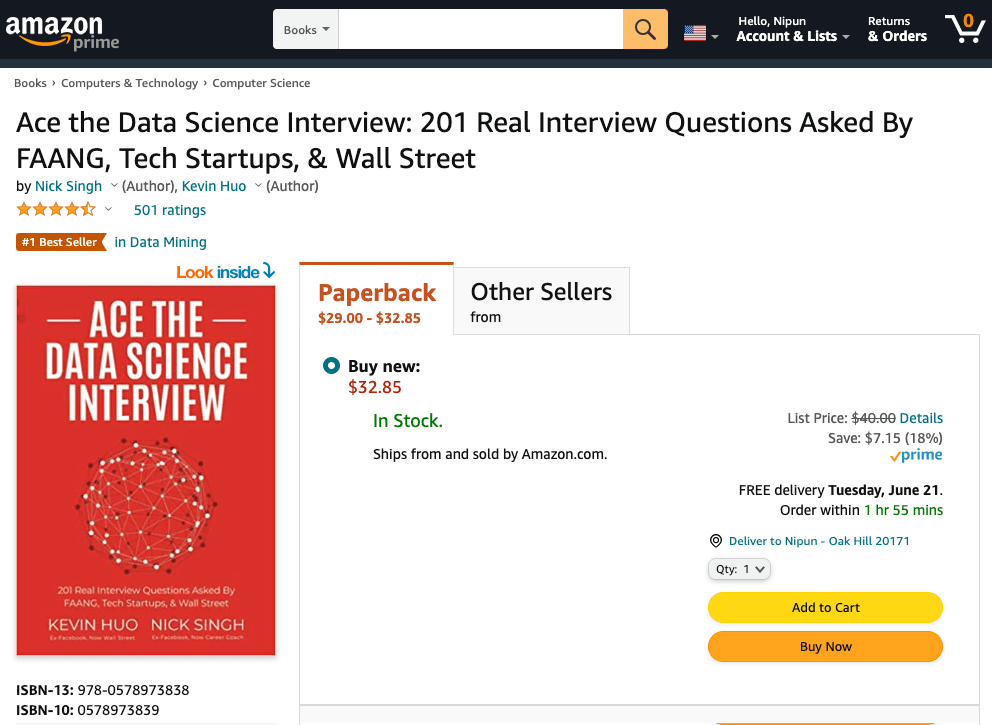
You can also enroll in my video course, Ace the Data Job Hunt, which covers the resume, portfolio project, cold email, and behavioral interview aspects of landing your dream job in data!

And finally, practice for SQL & Data Science Interviews on DataLemur! There are hundreds of real SQL, Statistics, and ML interview questions on there!
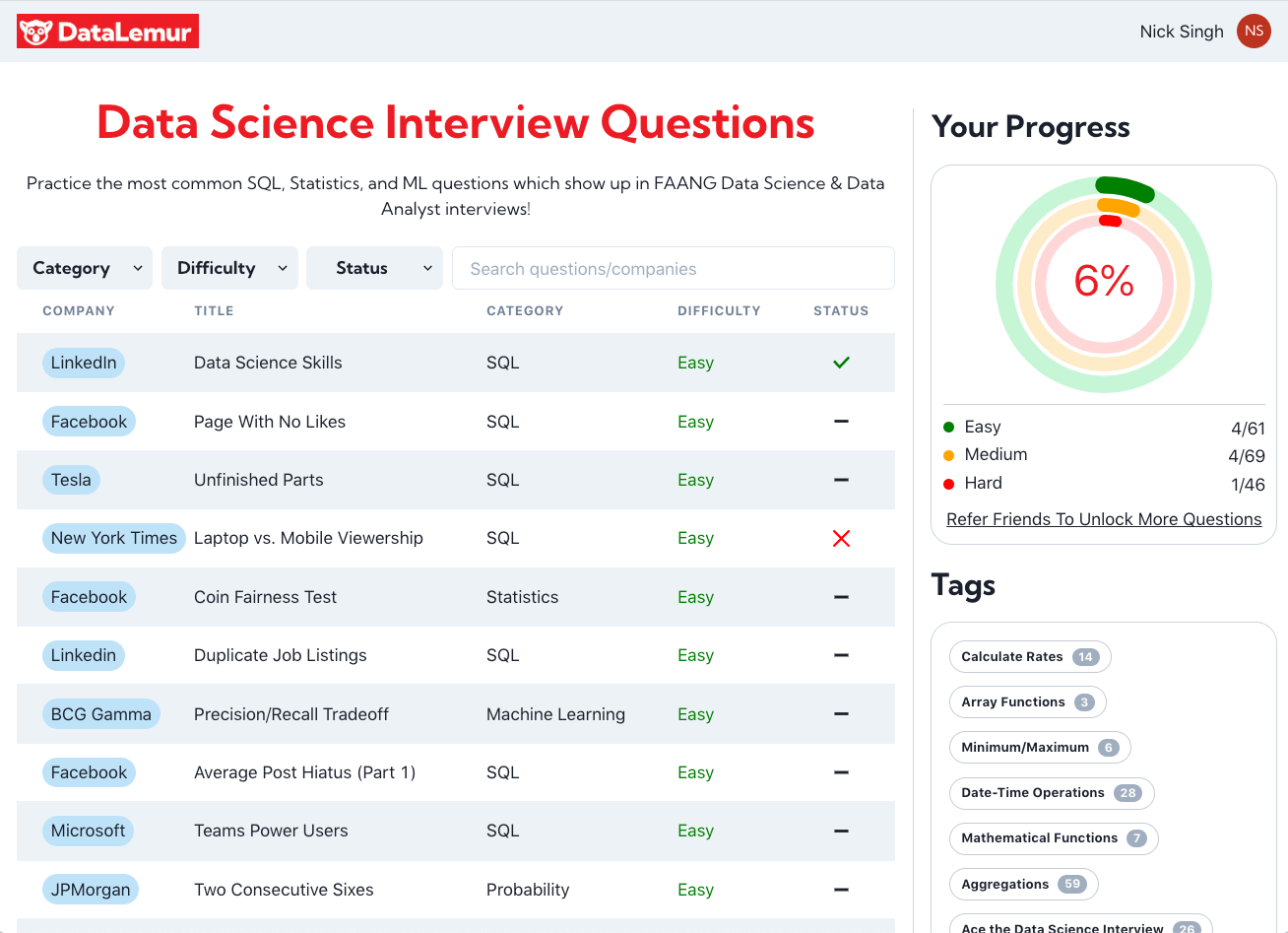